A Review of ANCOVA Model
A simple review of ANCOVA model based on the workshop by Naitee Ting.
Recall ANOVA
Look this picture (by Naitee Ting):
And keep this picture in mind:
ANCOVA
General Summary
ANCOVA is an alternative parameterization of the dummy-regression model, employing deviation-coded regressors for factors and expressing covariates as deviations from their means. The ANCOVA model can incorporate interactions among factors and between factors and covariates.
In ANCOVA, an ANOVA formulation is used for the main effects and interactions of the qualitative explanatory variables (i.e., the factors), and the quantitative explanatory variables (or covariates) are expressed as deviations from their means.
Neither of these variations represents an essential change, however, for the ANCOVA model provides the same fit to the data as the dummy-regression model. Moreover, if tests are formulated following the principle of marginality, then precisely the same sums of squares are obtained for the two parameterizations.
Technique Details in Clinical Trials Setting
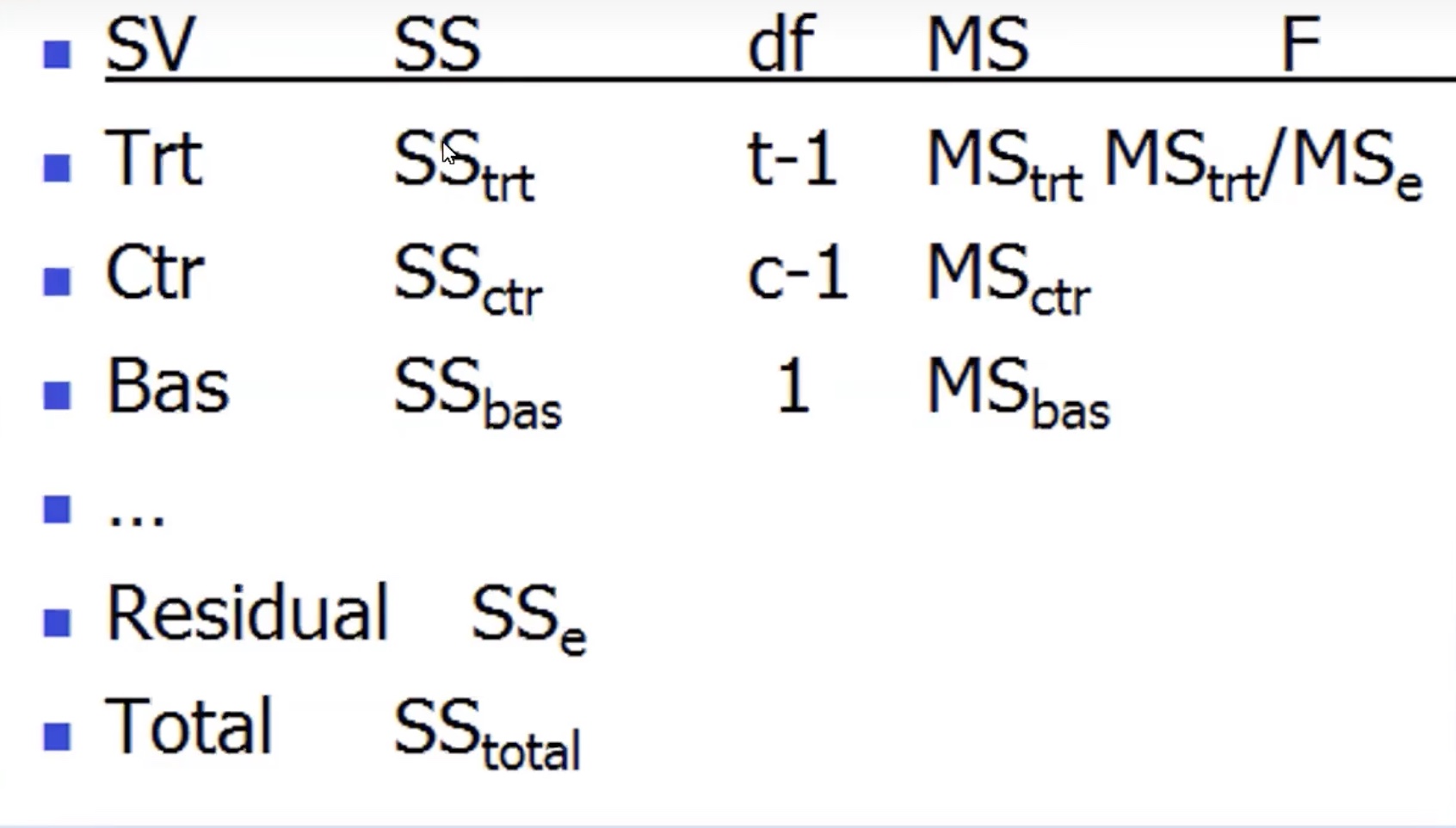
It corresponds to the below model:
\[ Y_{ijk}=\mu+\tau_i+\gamma_j+\beta X_{ijk}+\epsilon_{ijk} \]
where \(\mu\) is the overall mean; \(\tau_i\) is i_th treatment effect; \(\gamma_j\) is j_th center effect; \(X_{ijk}\) is the corresponding baseline; \(\epsilon_{ijk}\) is the residual error;
In the modeling process, statistician adds Covariates terms like baseline ("BAS" in the table above) into the ANOVA model. It will: - takes away some SS; - reduces SSE - make it esier to have a larger F-value and reduces P-value. It will make the model more powerful!
Chang from Baseline?
If we want to model change from baseline using the model above, things will change.
Suppose the observed treatment effect at week12 is \(Z_{ijk}\); baseline is \(X_{ijk}\).
There the change from baseline will be \[ Z_{ijk}-X_{ijk} \]
Model 1: change from baseline Let the change from baseline effect is modeled as \[ Y_{ijk}=Z_{ijk}-X_{ijk}=\mu+\tau_i+\gamma_j+\beta X_{ijk}+\epsilon_{ijk} \]
Model 2: week12 The new ANCOVA model for observation at week12 will be \[ \begin{aligned} Z_{ijk} &=Y_{ijk}+X_{ijk} \\ &= \mu+\tau_i+\gamma_j+(\beta+1) X_{ijk}+\epsilon_{ijk} \end{aligned} \]
From the above, why can we include the baseline covariate in the model?
- introducing baseline covariate into the both models above will have no impact on the inference on treatment effect \(\tau_i\);
- if \(X_{ijk}\) is continuous, it is actually a regression;
- introducing the baseline into the model can adjust for potential imbalance;
- help us reduce sum of residuals.
ICH E9 declares that we can include baseline, treatment and stratification factors into the analysis model in Phase III studies.
FDA Recommendations
https://www.bassconference.org/tutorials/BASS%202019%20Rubin.pdf
- Sponsors can use ANCOVA to adjust for differences between treatment groups in relevant baseline variables to improve the power of significance tests and the precision of estimates of treatment effect.
- Sponsors should not use ANCOVA to adjust for variables that might be affected by treatment.
- Even when the ANCOVA model does not closely approximate the true relationship between the outcome and the covariates, the probability of type I error is still maintained at the nominal level, and therefore misspecification of the relationship between the outcome and the covariates will not invalidate the results.
- The sponsor should prospectively specify the covariates and the mathematical form of the model in the protocol or statistical analysis plan. When these specifications are unambiguous, FDA will not generally be concerned about the sensitivity of results to the choice of covariates because differences between adjusted estimators and unadjusted estimators of the same parameter, or between adjusted estimators using different models, are random.
- Interaction of the treatment with covariates is important, but the presence of an interaction does not invalidate ANCOVA as a method of estimating and testing for an overall treatment effect, even if the interaction is not accounted for in the model.
- Even when the outcome is measured as a change from baseline, the baseline value can still be used advantageously as a covariate.